Learning Analytics: 3 Challenges and Opportunities
March 3, 2021
Keywords: educational systems, educational technology, learning analytics, organiational systems
Target readers: general audience
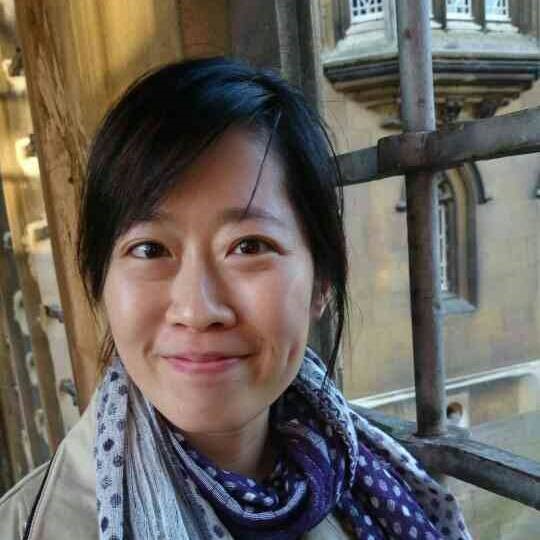
Author: Yi-Shan Tsai
Position: Lecturer in the Centre for Learning Analytics at Monash (CoLAM) at the Faculty of Information Technology, Monash University. Yi-Shan’s research aims to enhance learning, teaching, and educational environments with the adoption of digital and data technologies
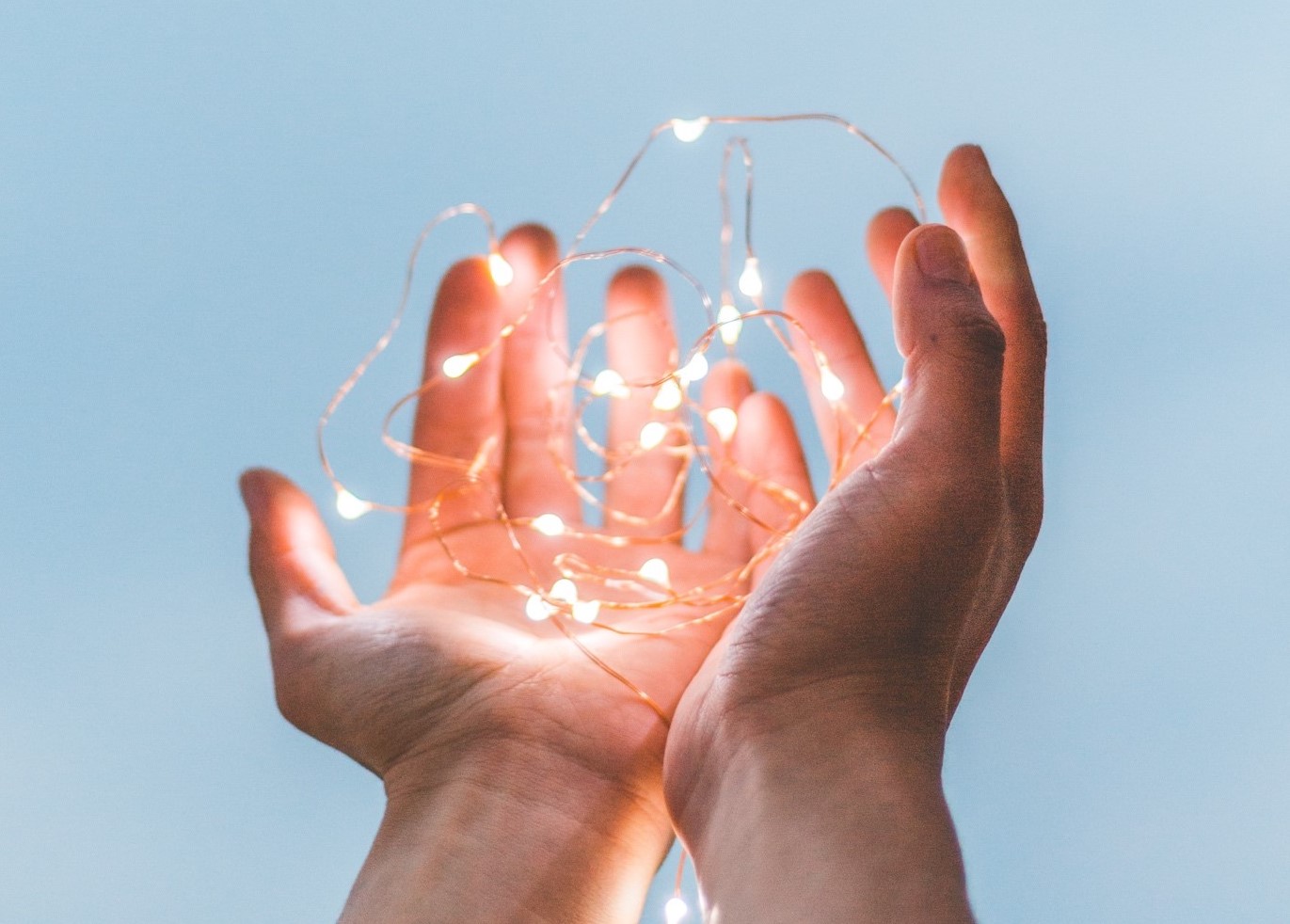
Photo by Diego PH on Unsplash
Learning Analytics: 3 Challenges and Opportunities
What are learning analytics?
Learning Analytics (LA) are commonly defined as “the measurement, collection, analysis and reporting of data about learners and their contexts, for purposes of understanding and optimising learning and the environments in which it occurs”. In essence, LA analyses and uses data trails produced by learners in learning environments to generate information about learners’ progress and learning patterns. Such information can further be used as a basis for prediction, intervention, personalisation, and adaptation, such as suggesting learning tips or materials and redesigning curricula. I made this video to give a three-minute introduction to learning analytics.
Why learning analytics?
Over the past two decades, the gross enrolment in higher education has more than doubled. A forecast of the global enrolments in higher education expects an increase from 214.1 million enrolments in 2015 to 594.1 million by 2040 (approx. 6,451 enrolments per 100k inhabitants). As higher education transforms from an elite system to a universal system, the question of how to meet the needs of a diverse student body and maximise opportunities to excel for individual students is one of the biggest challenges facing higher education today.
We have heard from different stakeholders in the past regarding drivers for adopting learning analytics (see the SHEILA project for details).
In general, institutional leaders are particularly concerned about institutional performance, which is commonly defined by metrics including student learning performance, teaching excellence, student satisfaction, and student retention. For example, one institutional leader told us:
“We want to focus on the learner experience, and we want to improve learner outcomes… If we can identify and flag up students that are struggling in their studies as early as possible, it might be a case that we keep them, we retain them, but also, we’re able to provide them with better support.” – Institutional leader
With near real-time data about learning engagement (e.g., trace data and social interactions) and other activities related to the measurement of key performance indicators, managers will be able to make better informed decisions to enhance the overall educational environment, such as timetabling, resource allocation, and programme structuring.
In contrast, teachers perceive learning analytics as useful in so far as providing an overview of students’ learning progress and some form of ‘user feedback’; that is, learner interactions with teaching methods and materials. The main interest is in improving the support for students and teaching quality. For example, common questions in the minds of teachers include: Did students watch the videos on topics X and Y? Do students struggle to understand key concept Z? Do students find the learning materials useful? A teacher commented:
“The existence of data and the existence of the tools to look at the data is really useful for the development of the course because you can reflect on what works, what doesn’t. What should I keep doing, what do I need to change?” – Teacher
Well-designed Learning analytics appeal to students particularly in aspects of personalised learning, quality feedback, and self-regulated learning. Students today come from various socio-cultural, economic, and educational backgrounds. Some students have special needs, some require language supports, some lack threshold knowledge that bridges subjects, and some may be juggling family and job responsibilities. Knowing where they stand in a learning journey and how to reach desired learning goals effectively is of the greatest interest to students. For example:
“I’m always curious about which areas I’m struggling in and which areas I am doing better in. If I had a profile of my learning then I could maybe spend more time revising the areas I’m struggling with. It would be a better way to spend my time.” – Student
Challenges and actions
Clearly, there is great potential of learning analytics in supporting decision-making related to learning, teaching, and educational management. However, applications in the real-world context remain small in scale due to various challenges concerning stakeholder engagement and buy-in, weak pedagogical grounding, ethics and privacy issues, and resource demand. As a result, the field of learning analytics is yet to establish the value and impact of learning analytics on the improvement of learning (see Ferguson & Clow (2017) and Viberg et al. (2018)).
Reflecting on the opening keynote by Professor Shane Dawson at the 10th International Learning Analytics & Knowledge Conference (LAK20), there is still a lot left for the field to work on in terms of ‘optimising learning’. Moving forward, there are a few areas that require our special attention:
1.Educational systems are complex
Challenges with learning analytics adoption have been observed in tensions that occur in a complex organisational network where individual agents have their own agenda (as also demonstrated above). While some people in an institution may see learning analytics as a potential means to innovate and adapt to a changing environment, others see the need to retain organisational efficiency and accountability. These conflicts, if unresolved, can be a force pulling the adoption of learning analytics backwards, resulting in stagnation or discontinuity of the adoption.
What it looks like in reality is that learning analytics may require new forms of infrastructure, system processes and expertise, which is disruptive to institutional stability and processes. It is thus important to have key leadership that can embrace the tensions, mobilise resources, establish a governance procedure, and importantly, align learning analytics solutions with existing problems that require solving, i.e., a problem-driven rather than a data-driven strategy.
2. Communication is key to a shared vision
The educational system is a complex network in which power is unequally distributed among stakeholders. As a result, teachers tend to find themselves ‘caught in between’, struggling to fulfil expectations from both managers and students while lamenting the loss of academic autonomy. On the other hand, students find themselves being excluded from the process of decision-making that is based on data about them.
In cases where policy procedures about using student data for learning analytics is in place, ineffective communications often result in the persistence of information asymmetry and mismatched expectations about how learning analytics should be used and how responsibilities are defined. Thus, establishing communication channels with different stakeholders is imperative to cultivate a shared vision and a sense of ownership over learning analytics. For example, a co-design approach has been advocated by researchers.
3. Learning analytics cycles need to be action-oriented
The concept that learning analytics is about learning should ground every decision we make during the design and implementation process of learning analytics. A key question that we should always ask is, what will change and for whom? Designers need to regularly check the linkage between analytics and action so as to ensure the usability and usefulness of learning analytics in classroom practice. Similarly, being able to extract information about learners’ behaviour and dispositions is not good enough. Researchers should seek to situate the data-based insights in learning and teaching situations. Instead of only asking what the data can tell us, go one step further and ask how the obtained insights can help learners and teachers.
Learning analytics are essentially a feedback process in which information needs to feed up to desired goals, feed back on where students currently are, and feed forward on steps to improve. Thus, learning analytics should never be only about feeding back. Teachers should seek to suggest ways for students to improve when providing learning analytics to facilitate self-regulated learning. Students, on the other hand, need to develop reflective skills that allow them to translate data into action.
2021 marks a new decade of opportunities for learning analytics. Where we are heading will depend on where we choose to start the journey. As we embrace the opportunities to better support students with data-based insights, it is important to pay attention to relevant socio-technical challenges and have a plan to address these.