Partnering with educators to take advantage of tools that predict student academic performance
August 22, 2021
Keywords: learning analytics, Online Teaching, Predictive Analytics
Target readers: researchers, leaders, educators
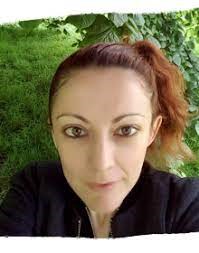
Author: Christothea Herodotou
Christothea Herodotou is an Associate Professor at the Open University UK with research interests in predictive learning analytics, citizen science, and mobile learning. She led the evaluation of the Early Alert Indicators project that enabled the adoption and use of predictive learning analytics across the Open University.
Or email her: christothea.herodotou@open.ac.uk
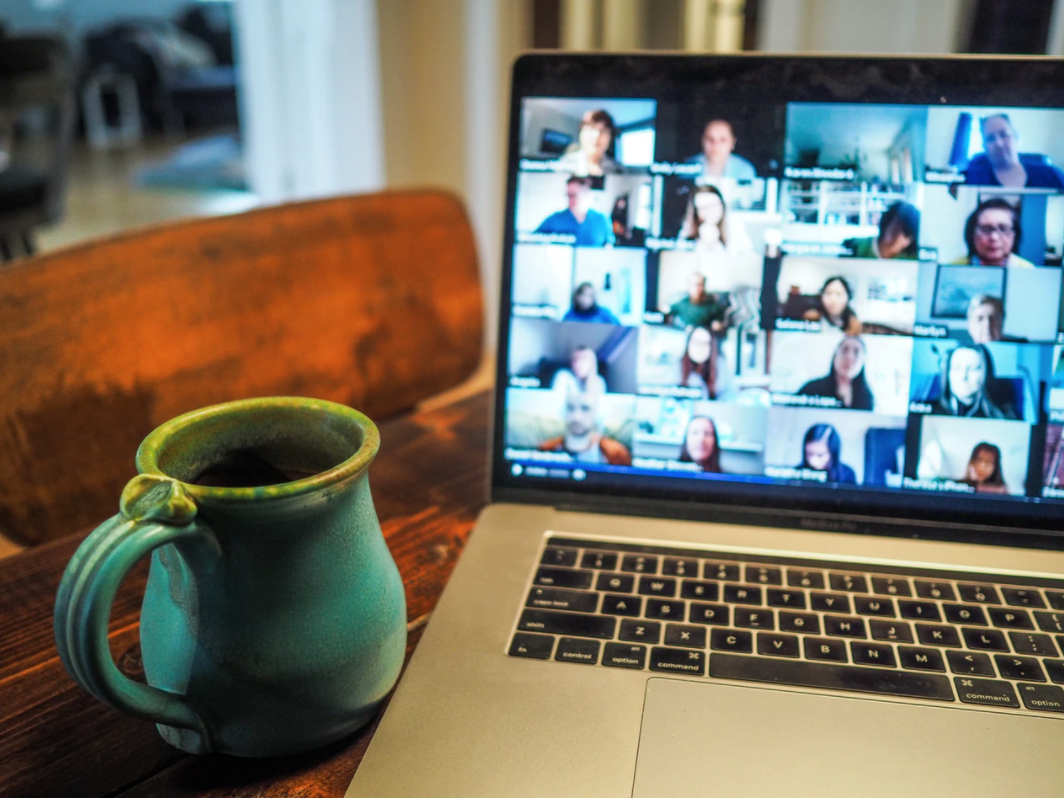
Photo by Chris Montgomery from Unsplash
Partnering with educators to take advantage of tools that predict student academic performance
In an earlier blog post, I told the story of Emma. Emma was an engineering student who appeared to perform well at her studies, but would have failed due to an upcoming pregnancy. Using predictive analytics, her online teacher identified a problem and intervened in time.
Emma’s story is far from unique. Over the years, teachers shared stories with me of how a predictive analytics system that forecasts their students’ future learning outcomes — in particular whether they are going to submit their next assignment — helped them to stay “on top” of their students. They gained insights otherwise not accessible to them because their students were studying at a distance.
Engaging teachers with predictive learning analytics is not straightforward. Some teachers devised their own ways of monitoring students and their progress. For example, by keeping their own records or checking on whether a student has submitted an assignment. Others rely on student support teams to identify and support students at risk of failing.
Asking teachers to use predictive learning analytics requires a change in teaching practice and more importantly a change in the way of thinking and acting as a teacher.
How can we change established ways of teaching to take full advantage of predictive analytics tools? How can we embed innovation in teaching in ways that teachers acknowledge its value?
Stakeholders often resist change. In higher education, researchers attribute resistance to change to factors such as the inertia in established organizational structures and staff with long-lasting positions.
Our work at the Open University UK demonstrated that for an innovation to become “business as usual” requires considerable time and effort. Working with teachers for more than four years, we learnt how to design innovations with teachers. We learnt what the actions are to make the journey, from introduction to systematic use, a less bumpy one.
I will share some key lessons we’ve learnt that I hope will help you introduce predictive learning analytics to teachers at your institution.
Lesson 1: Make teachers part of the research and design team
Inform the teaching community about the upcoming initiative to design and pilot a system that predicts students learning outcomes. A research team should also pay a group of teachers (ideally a group of them coming from different Faculties) to join the research team. They should make clear that the intention is to design a system that responds to teachers’ needs and requirements. The process of design should be participatory in nature; teachers are those who can share insights from the teaching practice and ensure that what is designed responds to their context, acknowledges challenges and functions in ways that can improve the teaching practice.
Lesson 2: Produce evidence of whether predictive learning analytics work for teachers and students
Evaluate the impact of using predictive learning analytics on students’ performance and the teaching practice. A research expert can facilitate implementation and data analysis, and sharing findings about the effectiveness of the system. Studies should not only be driven by project objectives, but also inspired by teachers’ reactions to the idea of using a predictive system. For example, one of the studies we conducted compared different ways of monitoring students’ progress, in particular predictive analytics to other practices, as a means to respond to teachers’ queries about whether the proposed system is a better way of monitoring students than other practices.
Lesson 3: Communicate the unique selling points of predictive learning analytics as…
…a consistent approach to monitoring students
The fact that each teacher may have a different way of monitoring students means that some students may have a more supportive teacher than others. For instance, a more proactive teacher may be able to identify and support a student early on. In contrast, a teacher who relies on student support teams may only find out a student was struggling after they fail. A research team should communicate to teachers that predictive learning analytics is a unifying approach to monitoring students to help ensure that all teachers have access to the same type of student information.
…a systematic approach to checking on students’ progress
Some teachers may be aware of what students are doing and how they are progressing without the use of a system. Yet, there may be unexpected cases of students that may fail if a teacher is not systematic in checking on new predictions when these are produced (in our case once a week). Even a well performing student may be at risk of failing (read the story of Emma) when their personal circumstances change. Checking on new predictions can provide insights that a student has not declared, and which a teacher is not aware of.
…a call to action rather than a self-fulfilling prophecy
Some teachers may view predictions as a self-fulfilling prophecy, that is, predictions will be confirmed no matter what the teachers’ response to a prediction is. Emphasize to teachers that predictive analytics are a call to action and that they are most likely to become a self-fulling prophecy when a teacher fails to take action early on to support and help a student to submit their next assignment.
…insights BEFORE a student fails
To assess students’ progress, some teachers may rely on whether a student has submitted and passed their first assignment. This is viewed as an indicator of whether a student is doing well. While this may be a good proxy of student progress and performance, this information is available rather late and after a student has already failed their first assignment.
Researchers have showed that failing to submit a first assignment is likely to lead to failing the course. Therefore, teachers should have access to information about students before they submit their first assignment. Predictive analytics inform teachers BEFORE an assignment outcome is produced. When teachers check analytics well ahead before the assignment submission deadline, they can allow students time to work towards an upcoming assignment, submit and eventually pass it.
To sum up, in our experience from four years of embedding predictive analytics in a distance and online university, teachers are most likely to see the value of such tools for their teaching when they are meaningfully involved in the process of designing and piloting such a project, while at the same time important messages are clearly communicated with them.
Online teaching and learning are of course now the sudden experience of millions of students and teachers due to the pandemic, leaving many teachers feeling like they are “flying blind”, with less insight into how their students are doing.
Our experiences demonstrate convincingly that “online students” ≠ “invisible students” — in fact, they are digitally more visible — if your institution has built the human-centred analytics ‘glasses’ to see and support them.
Our experiences also show that this is a learning journey, and I hope that sharing what we’ve learnt helps you map your path. Should you want to find out more recommendations about how to implement predictive learning analytics at your organisation, you may like to read this paper.
To learn more…
Herodotou, C., Rienties, B., Hlosta, M., Boroowa, A., Mangafa, C., & Zdrahal, Z. (2020). The scalable implementation of predictive learning analytics at a distance learning university: Insights from a longitudinal case study. The Internet and Higher Education, 45, 100725.
Herodotou, C., Hlosta, M., Boroowa, A., Rienties, B., Zdrahal, Z., & Mangafa, C. (2019). Empowering online teachers through predictive learning analytics. British Journal of Educational Technology, 50(6), 3064-3079.
Herodotou, C., Rienties, B., Boroowa, A., Zdrahal, Z., & Hlosta, M. (2019). A large-scale implementation of predictive learning analytics in higher education: the teachers’ role and perspective. Educational Technology Research and Development, 67(5), 1273-1306
Herodotou, C., Rienties, B., Verdin, B., & Boroowa, A. (2019). Predictive learning analytics ‘at scale’: Guidelines to successful implementation in Higher Education based on the case of the Open University UK. Journal of Learning Analytics, 6(1), 85-95.