Leveraging the Potential of Learning Analytics in Higher Education Requires Inclusive Policies and Practices
March 18, 2021
Keywords: Data Ethics, Ethics, Higher Education Policy, learning analytics
Target readers: Higher Education Leaders, Learning Analytics Researchers
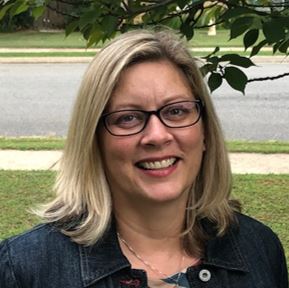
Author: Carrie Klein
Position: Senior Fellow, Future of Privacy Forum’s Youth & Education Team and Affiliate Faculty, George Mason University’s Higher Education Program, USA
Carrie’s research works to illuminate the intersection of analytics and surveillance technologies, organizational structures, policies, and practices, and individual decision making and agency with the goal of improving equitable student and institutional outcomes.
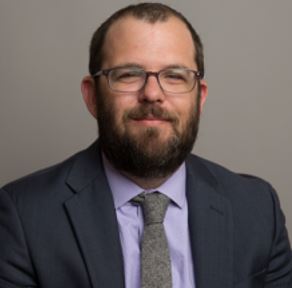
Author: Michael Brown
Position: Assistant Professor, Iowa State University, USA
Michael’s research focuses on the development of instructional activities, curriculum, and educational technology in undergraduate education with a focus on how institutions use data assets as part of student success initiatives and how instructional reforms using digital technologies can expand access to Science and Engineering fields.
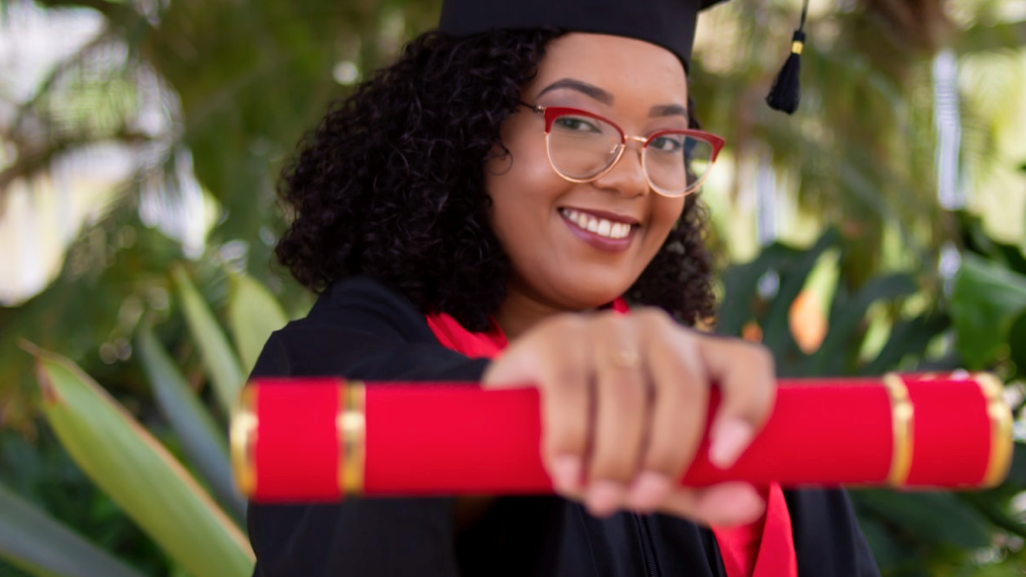
Photo by felipegregate on Unsplash
Leveraging the Potential of Learning Analytics in Higher Education Requires Inclusive Policies and Practices
Policy making in US higher education lags technology implementation. Institutions, which have made major investments in learning analytics informed strategies, have made few updates to their associated data privacy policy literature. In the summer of 2019, we conducted a policy discourse analysis of post-secondary institutions’ data privacy policies to understand how institutions frame student data rights and responsibilities and the implications of that framing given the lag between policy and practice. The results were relatively uniform and striking.
We found that privacy and data policies tend to portray antiquated conceptions of data, not as dynamic systems, but as static records. Further, while data were perceived to be valuable (notably, there was a focus on institutional risk associated with the collection and use of data) changes to a student record were treated as costly and burdensome.
Policies also positioned a wide array of individuals with legitimate education interest in student records in order to support the universities’ function (for instance, data sharing with third parties), with little regard for how they might use or commercialize student data.
Finally, and most importantly, institutional policies assume that students are informed agents; yet, fail to provide clearly communicated student rights or institutional responsibilities beyond stock, federally mandated statements. As a result, student data are increasingly collected, used, and shared by institutions, within and beyond learning analytics systems, with little centering of the student within the policies that govern student data practices.
To address this disconnect, we have been thinking about what a student-oriented approach to institutional governance might look like when data about students and their contexts proliferate. We were both student success practitioners before we became learning analytics researchers, and our primary interest is in how we can use the systems of institutional governance to build systems that promote student input and control over their representations and outcomes.
In our thinking, we draw upon Taylor’s (2017) framework for data justice but situate it within the context of higher education, which Slade and Prinsloo (2014) and Prinsloo and Slade (2017) have argued has an ethic of care that should guide work with students and their data. Taylor’s data justice framework includes three pillars (p. 9):
- Visibility (including individual access to representation and informational privacy)
- Engagement with technology (including individuals sharing in data’s benefits and autonomy in technology choices)
- Non-discrimination (including an individual’s ability to challenge bias and to be free from discrimination)
Data justice centers the individual experience in data systems and contrasts with traditional approaches to data use in higher education, which centers institutional structures and practices over student perspectives and needs. For example, the construction of student data warehouses, which are often opaque, institutionally controlled, and managed in part through classification algorithms (whose primary purpose is to discriminate among observations in the broadest sense) work to meet the needs and priorities of the institution. While students may benefit from data use, they are not active or represented in the policies or practices that govern use of their data.
Drawing from Taylor’s data justice framework and the pioneering work by Prinsloo and Slade on ethical and care-based practices in learning analytics, we argue learning analytics researchers, student success practitioners, and faculty should work together to establish an inclusive approach to policies that govern learning analytics data use. This approach is predicated on three key principles — establishing equitable systems, on ensuring transparency and trust, and on empowering informed agency — and works not only to advance the pillars of data justice for individuals within those systems, but also creates the conditions to encourage a more just and care-based use of student data. Table 1 at the end outlines the relationship between the principles, technological and organizational responsibilities, and individual abilities and rights for inclusive learning analytics use in higher education.
An inclusive approach works to ensure equitable systems through actively accounting for and redressing any bias or discrimination in technological systems (i.e., learning analytics systems) or organizational systems (i.e., student learning and success work). This approach recognizes that information asymmetry and power dynamics at play in higher education socio-technical systems not only exist, but must also be addressed and remediated.
One way to identify and disrupt and address instances of bias and discrimination is through audits of data systems (and underlying algorithms) and associated organizational structures and policies. This work should be done through the life-span of any data system and be conducted by an interdisciplinary team, to include students, student advocates, and equity experts in addition to data administrators, technologists, and researchers. Further, students must have the ability to report and challenge any instances of bias or discrimination and have clear pathways (like responsible policies) to do so.
Those pathways are reliant on ensuring transparency and trust in data representations and organizational policies and practices. Tied to the tenet of visibility, institutions must make clear to students how they are represented by their data and how their data are used — by whom, for what purposes, and for how long. Further, learning analytics data should both accurately represent student realities and responsibly inform student interventions. These technological and organizational obligations are dependent upon communication.
For students to be able to rely on how their data is used, data visualizations and interventions, and organizational policies and practices, must be relayed in relatable language and in spaces students use and make clear what rights students have related to their information. Too often, institutions communicate policies to students in overly complex or jargon-laded legal language and data visualizations are inaccurate, incomplete, or not fully relevant to student perspectives. To encourage inclusive policies and engender trust, students should know they can rely on accurate data and be involved in determining how they are represented in systems. Data governance teams focused on data use and policy development should be interdisciplinary (again, including students, student advocates, and equity experts) and should work to build student trust through transparent data and communication.
Finally, to fully engage with technology and share in its benefits, organizations must work to empower students as informed agents. This requires that the institution create policies and opportunities that allow students to engage with their data – beyond notice and consent. Students be actively included in the development and implementation of tools that collect their data and in how those data represent them and inform their choices. However, agency is dependent upon policies that encourage data-based education at all levels of the organization. Data literacy should be included in the general curriculum, so students have basic knowledge, language, and skills to understand how their data are used – both within higher education and beyond. Faculty, advisors, administrators and other users of student data should also participate in ongoing professional development to understand issues of data bias and discrimination, so that they can put that learning into practice. They should work to make visible the use of data in the general curriculum, in syllabi, in advising appointments, and communications. They should also work to ensure that students data rights and protections are reflective of modern data systems and use. These actions can help empower students to be informed, active agents in their digital representations and future outcomes.
It would be easy as learning analytics scholars and practitioners to say that the above objectives are beyond the scope of our work. That governance is the realm of faculty and educational leaders, and that the way our data products and tools are used should be shaped by student success practitioners. This elision of responsibility ignores how our work — the making of databases and the ways in which they are put to use — is an act of institutional worldbuilding (Benjamin, 2017). If the work that we do has the potential to constrain individual autonomy, choice, or opportunity, we have a moral responsibility to actively engage as part of the institution in shaping use and implementation of learning analytics products.
Who better to provide technical insight and to chart potential harms? Who else should be responsible for dealing with the potential consequences of our work than its authors? As Gary Hall (2016) notes in The Uberfication of the University, our field has fostered significant interest in personalization through student data use among senior academic leaders. As a consequence, we have a responsibility to cultivate that interest towards an ethic of care built on just, humane technologies.
Table 1. Principles for Inclusive Learning Analytics Use in Higher Education
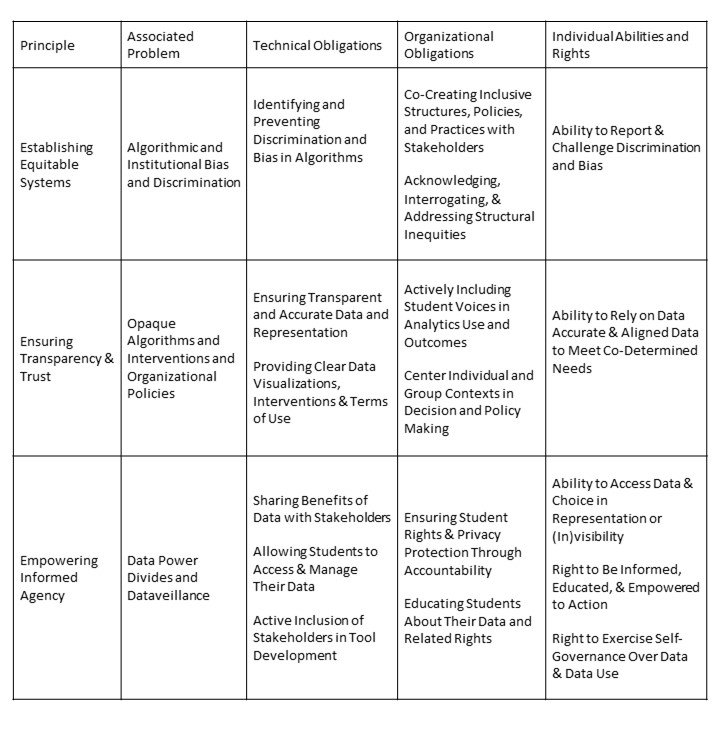
Learn more…
Brown, M., & Klein, C. (2020). Whose data? Which rights? Whose power? A policy discourse analysis of student privacy policy documents. The Journal of Higher Education, 91(7), 1149-1178, 10.1080/00221546.2020.1770045
Hall, G. (2016). The Uberfication of the university. Minneapolis, MN: University of Minnesota Press.
Slade, S., & Prinsloo, P. (2014, October). Student perspectives on the use of their data: Between intrusion, surveillance and care. In Proceedings of European Distance and E-learning Network Research Workshop (Vol. 27, p. 28).
Taylor, L. (2017). What is data justice? The case for connecting digital rights and freedoms globally. Big Data & Society, 4(2), 2053951717736335.
Prinsloo, P., & Slade, S. (2017). Big data, higher education and learning analytics: Beyond justice, towards an ethics of care. In Big data and learning analytics in higher education (pp. 109-124). Springer, Cham.